Jins Antony and Grace Janilet from Ayur in Kerala are in a race against time, seeking a cure for their firstborn, Jion. They are acutely aware that unless medical science expedites its efforts to find a miracle drug for their child’s worsening condition, he will die soon. Jion, 10, suffers from Duchenne muscular dystrophy (DMD), a genetic disorder characterised by progressive muscle degeneration and weakness. “This condition arises due to alterations in a protein called dystrophin, which helps maintain muscle cell integrity,” says Antony, 42, who runs a paint shop. DMD can affect all kinds of muscles, including the diaphragm―the muscular partition separating the thorax from the abdomen, and which aids breathing. And, that makes it fatal. “Currently, there is no definitive cure for this rare disease,” says Antony, adding that time is running out for Jion, “as Indian DMD patients rarely survive beyond 20 years”.
In the past, Jion, like any other kid his age, would run and play around. But these days, he struggles to walk without support. His eyesight, too, has diminished in recent years. At school, while other children play, he sits and watches them. He does not join them for fear of falling and breaking his bones. He still rides his bicycle, but is unsure if he can continue his favourite activity for long.
DMD is caused by a mutation in a gene that encodes the dystrophin protein, and is located in the X chromosome. Since the gene is tied to the X chromosome, DMD usually affects individuals assigned male at birth―since they have XY chromosomes, they receive only one X chromosome from their mothers and therefore have no other functioning gene to compensate.
DMD symptoms usually show up by the age of three, but children are usually diagnosed at the age of six. Jion was diagnosed with DMD only when he was seven. “We were unaware of this disease until then,” said Antony. “My wife and I did not sleep the first two months after we learned about the test results.”
Steroids are the mainstay of DMD treatment today. Gene-based therapies like exon-skipping to produce usable dystrophin protein are available, but are too expensive. For instance, exon-skipping therapies, such as deflazacort, at its approved dose, can cost approximately Rs 7.5 crore per year. Antony has already shelled out a fortune for Jion’s treatment in the last three years. With no cure and the long-term expense and effort involved, caring for a loved one with DMD can take a toll on any family with limited means. The thought in itself can be daunting and depressing. In July 2023, a family of four, including two children, died by suicide in Kerala’s Malappuram after the childen were diagnosed with DMD. Antony and Grace, too, were depressed in the initial months of Jion’s diagnosis. “However, after a phase of depression and lamentation, my wife and I decided not to give up,” said Antony. “We face every day with the hope that the medical community will soon come up with an affordable drug for my son’s condition, before it is too late.”
The good news for many families like Jion’s is that there are several Indian companies working for accelerated and cheaper drug discovery with the help of artificial intelligence. One such company is Peptris―an eight-member virtual preclinical research firm that focuses on helping develop drugs for muscle-related rare diseases like DMD.
Drug discovery is a slow process, taking 10 to 15 years. It involves research, development and production of molecules, which are like keys that open a disease’s lock. So, labs are finding the right key among many for a specific disease or modifying an old key to fit a new lock. And, chemistry plays a vital role here because of its ability to decode molecular interactions. Many of the drugs developed in the last century come from molecules derived from natural sources like plants, bacteria and fungi. As the discovery and development of these molecules slowed, manmade molecules were brought in. But they are not enough.
In drug discovery, various strategies are employed to identify potential drug candidates. These may be broadly classified into three types: target-based drug discovery, phenotypic drug discovery and repurposing existing drugs. Target-based drug discovery involves understanding the pathophysiological (abnormal changes in body functions) basis of disease and identifying specific protein targets that play a role in the disease phenotype. Phenotypic drug discovery focuses on observing the effects of potential drugs on whole cells or organisms without prior knowledge of the target. The repurposing existing drugs strategy, the cheapest among the whole, involves evaluating drugs developed for specific therapeutic purposes for potential use in new disease areas.
Shridhar Narayanan, cofounder and scientific adviser of Peptris, told THE WEEK that in diseases like DMD, the startup is employing two of the above-mentioned strategies with the help of AI to create a potential drug. “One is to use artificial intelligence to find novel chemical molecules for particular targets, and second is repurposing, in which we examine already existing drugs for some of these disorders,” he said. “We have put a priority on finding some drugs that can be repurposed and be given to DMD-affected kids at the earliest.”
Riyaz Syed, founder of Hyderabad-based AI-first drug discovery company, Centella AI Therapeutics, said that other than muscular dystrophy, idiopathic pulmonary fibrosis and amyotrophic lateral sclerosis are other rare diseases for which research powered by AI is being used in target identification and target validation.
Time travellers
The drug discovery value chain is extensive, beginning with the phase of identifying and validating disease targets. These targets refer to proteins responsible for the disease. Once a target is identified and validated, the focus shifts to designing new drugs tailored to that target. “This involves starting with molecules and progressing to lead optimisation and the selection of preclinical candidates,” said Syed. “However, the initial design may not yield a viable drug, requiring multiple iterations and optimisation of various parameters, such as absorption, distribution, metabolism and excretion. This iterative process, known as the DMTA cycle (design, make, test, analyse), involves designing molecules for disease proteins, testing them in laboratory settings and analysing the results. Typically, this process involves seven to 10 iterations, depending on the complexity of the disease.” What AI does is to streamline this process by reducing the number of iterations and minimising human intervention, added Syed. “Instead of conducting numerous experiments, AI can predict which experiments are most likely to succeed in the laboratory, thereby accelerating drug development and reducing delays in delivering therapies to patients,” he said.
Shridhar, who has a postdoctoral degree in neuro-pharmacology and heads a not-for-profit organisation―Foundation for Neglected Disease Research―said that in the past, researchers would perform limited chemistry experiments and conduct high-throughput biology tests, which is essentially testing thousands of molecules at once for their efficacy against a specific drug target. “They would test compound libraries (collection of stored chemicals) synthesised for various purposes for each new target they identified. Now, with AI, things are different,” he said. “While you may focus on a specific protein, the number of chemical molecules you can explore is vast, running into billions. This increased chemical diversity, facilitated by AI, significantly boosts the chances of finding successful compounds. When it comes to biological assays, setting them up, validating them and screening millions of compounds is no small feat, both in terms of time and money. However, AI now allows for this to be done with larger compound libraries at a fraction of the cost and time.”
Shridhar has been part of teams that have created 18 clinical candidates for infections, diabetes, oncology, inflammation, pain and cardiovascular diseases. Interestingly, Peptris, which raised $1 million in a funding round in December, was formed as a result of a 'dad talk' Shridhar had with his cofounder Narayanan Venkatasubramanian, an IIT Delhi alumnus with more than two decades experience in system architecture and software development. “Our children attend the same school, which led to us to becoming family friends,” he said. “This connection gradually evolved, leading us to engage in discussions beyond school-related events. We began inquiring about each other's professions, interests and the latest developments in our respective fields, fostering a deeper understanding of each other's domains. So, it was our children who initially brought us together, and from there, the foundation for a novel idea was laid.”
Along with Shridhar and Venkatasubramanian, data science expert Anand Budni and algorithm expert Amit Mahajan form the core team of Peptris. Together they developed an AI and machine learning-based computational platform to rationalise and provide insights into experimental data in molecular biology and further make predictions to generate hypotheses for newer experiments to be done.
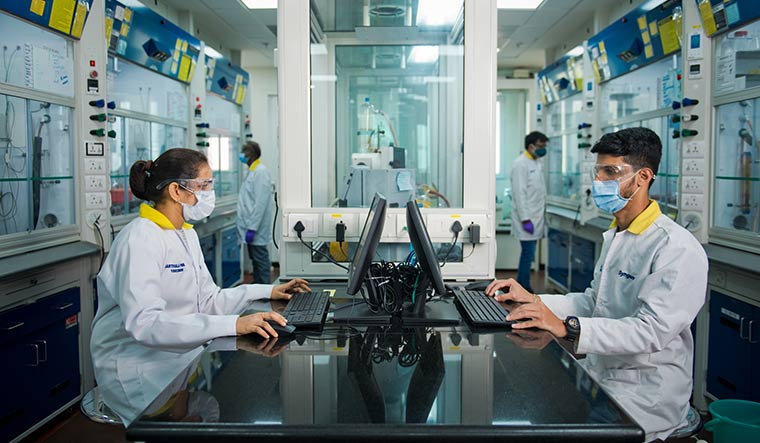
“We are now able to screen over a billion molecules essentially employing AI,” said Venkatasubramanian. “Instead of physically conducting tests, we can now perform these tests virtually. Over the last four or five years, the number of chemical entities, or novel chemical entities, has increased significantly. However, this abundance of information isn't easily searchable. For instance, if you want to find out which molecule would work for a specific protein, you would need to sift through a vast amount of data to provide the best possible results. So, the challenge lies in developing algorithms that can conduct such searches effectively.”
The software expert added that there is a need to represent molecules in searchable formats to determine the best matches or approximate matches for a given protein. Then, further analysis and testing would be conducted to validate the findings. “Essentially, it is like conducting a mini search within the vast chemical space available,” said Venkatasubramanian. “You could think of it as organising a vast collection of books in a library and cataloguing them in a specific way to facilitate easy retrieval. In this context, AI is akin to creating a catalogue for these molecules, making unique representations for each of them.”
Peptris has meticulously gathered millions of data points, encompassing structural details, annotations and properties data related to proteins, molecules and their interactions sourced from public databases. It now has the capability to accurately predict binding affinities in both protein-small molecule and protein-protein interactions. This essential capability is what they use to expedite drug discovery in different domains.
Syed pointed out that the efficiency that AI brings to the drug discovery process is invaluable. “Our generative algorithm produced approximately 15 molecules targeting a specific cancer type, and we synthesised them accordingly,” he said. “The success rate from these synthesised molecules was approximately 80 per cent, which is notably high compared with the traditional success rate of 35 to 50 per cent. Our method consistently achieves success rates ranging from 75 per cent to 80 per cent, demonstrating its efficiency in generating novel and patentable molecules. Subsequently, we synthesised these molecules in the laboratory and conducted tests. Among them, approximately three molecules exhibited superior activity compared to the standard drug.” Centella has a special focus on diabetes, inflammation and gastrointestinal infections, too. “We are working on four targets based on these areas,” he added.
Shining results
Syngene, founded in 1993 and headquartered in Bengaluru, is a leading provider of contract research services as well as integrated discovery, development and manufacturing solutions for various industries, including pharmaceuticals, biotechnology, animal health care, consumer goods and agrochemicals. Recently, Syngene made a significant breakthrough in combating pancreatic cancer by collaborating with Panbela, a US-based clinical-stage biopharmaceutical company, to streamline the production process for its pancreatic cancer drugs using AI technology.
Before partnering with Syngene, Panbela was manufacturing its small molecule candidate SBP-101 for pancreatic cancer using a complex 15-step synthesis process. On engaging Syngene for chemical development, chiral purity checks (essential for quality control of drugs) of early batches revealed a chiral purity of only 85 per cent, falling below regulatory standards.
Syngene revamped the synthesis approach with the help of Syn.AI, the Bengaluru-based firm’s exclusive platform tailor-made to streamline data-centric drug discovery. This helped in preventing the formation of undesirable intermediates, and led to the production of SBP-101 with a chiral purity of 98 per cent, albeit with 17 synthetic steps. Subsequently, Panbela tasked Syngene with reducing the number of reaction steps to expedite the drug's time-to-market while maintaining chiral purity. Syngene's chemists initiated the optimisation process, and eventually enabled the synthesis of SBP-101 in just six steps.
“This patent, which outlines a more concise synthesis method for SBP-101, offered numerous advantages, including faster manufacturing, expedited access to drug supply, expansion into new indications and the establishment of a scalable, efficient, and cost-effective manufacturing process to support future commercialisation efforts,” said Jennifer K. Simpson, president and CEO of Panbela.
Future is now
According to market research and consulting firm MarketsandMarkets.com, the global market for AI in drug discovery is estimated to reach $4.9 billion by 2028, showing a substantial increase from $0.9 billion in 2023.
Meanwhile, researchers like Shridhar attest that significant transformations have occurred in the drug discovery domain in recent years. Specifically, he highlights the disruptive impact of newer and advanced technologies like ‘click chemistry’, which offer a rapid, straightforward and adaptable approach to synthesising new molecules, thereby expediting the drug discovery process.
“What click chemistry has achieved is remarkable,” he said. “Over the past five to 10 years, there has been an exponential increase in the number of synthesisable molecules that can be produced with just two or three steps and lower energy requirements in a standard laboratory―all thanks to click chemistry. This advancement has resulted in a significant surge in the volume of data generated in the chemistry domain.” For example, in 2017, the largest available libraries contained fewer than a billion compounds, whereas now they contain approximately 43 billion to 44 billion compounds, he said. “This number continues to escalate rapidly, demonstrating the accelerated evolution of synthesisable and purchasable compounds, which in turn creates a superhighway for discovery processes,” said Shridhar.
Furthermore, researchers emphasise that the abundance of data leads to the emergence of more solutions in drug discovery. They also assert that they do not foresee AI replacing humans in fields such as drug discovery.
“The drug discovery process carries profound implications for life and death. We cannot entrust artificial intelligence with making decisions on our behalf,” said Syed. “At each stage, every action taken by artificial intelligence undergoes thorough scrutiny, meticulous analysis, and only advances to the next level with human intervention.”