The Burning Question is a column that tackles some of the biggest questions in the intersection of science, technology, geopolitics and culture that shape the world as we know it. The column will soon be expanded into a newsletter, and you can subscribe for free here. Subscribers will receive updates from the column via email, Telegram. Write to editor@theweek.in with comments, suggestions and questions.
In mid-19th century, when the Cholera epidemic affected Britain, a common perception was that the disease was transmitted and spread by a "bad air" or "bad smells" from rotting organic matter. That was challenged by an anaesthetist John Snow, who, after mapping deaths from the disease, noticed clusters among families who depended on a specific public water pump on Broad Street. As it turned out, the water was polluted by sewage from a nearby cesspit—a common phenomenon under most homes at a time when proper sewer systems were a premium. In addition to helping 'flatten the curve', this led to a 180° degree turn in how the disease and its progression were analysed by the medical fraternity. Since the Cholera plague, the world has lived through multiple global viral outbreaks. The Russian flu was reported in 1889, the Spanish flu made its appearance in 1918, the Asian flu arrived in 1957, SARS came in 2002, Ebola in 2014, MERS in 2015, and COVID-19 in 2019.
The novel coronavirus is markedly different from any of the pandemics that came before it, a phenomenon highlighted by researcher Silvio Pitlik in the US National Institute of Health (NIH) journal. First, there is the large number of asymptomatic cases—according to most statistics, almost 80-85 per cent infections don’t show any outward signs. Then there is the remarkably wide spectrum of clinical manifestations of the disease. There were reported cases of cardiac issues like myocarditis, myocardial ischemia, and myocardial infarction. There can be hepatitis, pulmonary embolism, encephalitis, acute renal failure, and Kawasaki Syndrome among teenagers. Add to all this uncertainty the rise of multiple variants, and the entropy of unprecedented state intervention measures like lockdowns, global travel bans, and a hitherto unparalleled scale of vaccine deployment.
This level of tumult makes mapping the pandemic—what Snow did with Cholera—a daunting task, especially in vastly heterogenous systems. In the Indian context, the most talked-about model is SUTRA, a data-centric approach to predicting the trajectory of the pandemic, developed by IIT Kanpur, and backed by the Union government’s Department of Science and Technology (DST).
Read SUTRA paper here
Says Manindra Agrawal, former deputy director of IIT Kanpur and co-founder of the model: “Take a parameter like the magnitude of spread of the disease. To quantify it, epidemiologists would use something like the Google mobility data and the population density of the region, combined with a general understanding of how people interact with each other. They will combine all that knowledge and come up with an estimate of the value of spread parameter. However, that is totally extraneous to what the data is saying. Our approach has been to simply use the data to infer the value of the necessary parameters.”
How SUTRA 'chases' the pandemic
Before getting deeper into the SUTRA model, a short primer on the evolution of modelling through the years is in order. At first, there were statistical simulations like the Gaussian distribution curve, where available data is fit into the function. Here, an exponential surge in infections at the start of the pandemic will be followed by a peak, and then a period of decay when state intervention, (acquired/induced) immunity, and other factors, all start playing their roles.
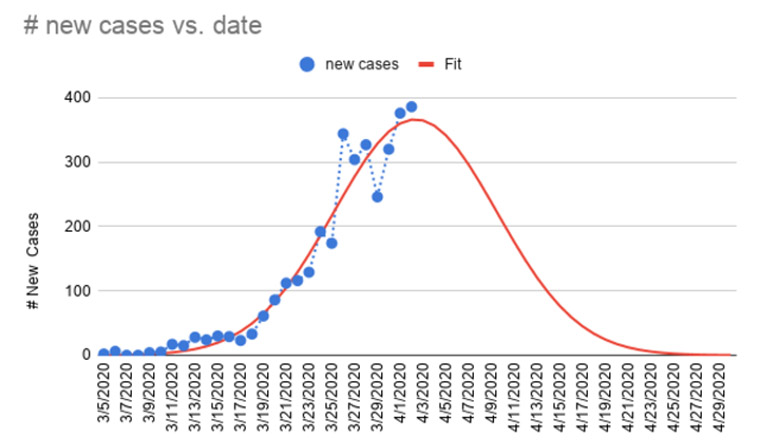
Gaussian curves by-and-large fit the coronavirus outbreak models across the globe, at least in early stages before variants started appearing. The limitations of such a model become apparent when we take into account its predictive capacity—when it comes to foretelling the trajectory of the pandemic, so that policies can be crafted around those approximations, the Gaussian model falls short.
Then came mechanistic simulations (with good performance stats when it comes to predicting respiratory outbreaks like SARS and MERS), the most popular being the Kermack-McKendrick SIR model that evolved around the time of the Spanish flu—from 1918 to 1920. SIR divides the population into three categories. First, there is the susceptible population (those at risk), denoted as S(t) at any point of time t. There is the infected populace (I), who are carriers of the disease and can infect the susceptible, and the removed (R), who have developed immunity to the disease (by whatever means) or died. The S category decays over time into I, and then into R.
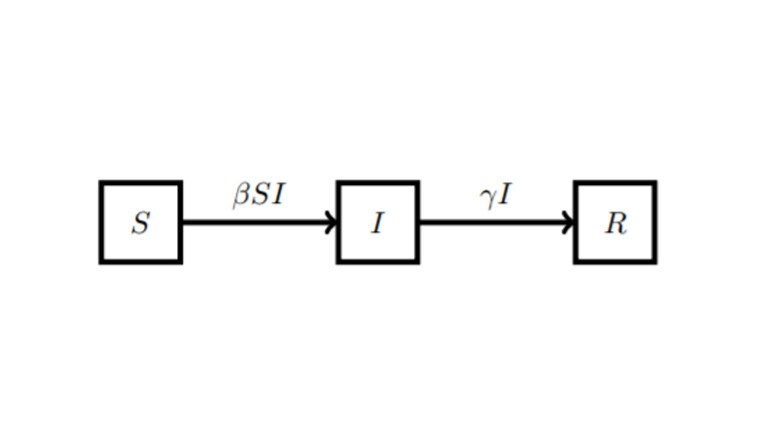
Two major parameters are involved in this model—β (beta) and γ (gamma). β is the probability of fresh infection arising out of contact between an infected and a susceptible person, thus deciding the velocity of movement from the S to the I category, and γ decides the rate at which infected persons move to the R category.
As further disease outbreaks arose, the SIR model was revised. When the Malaria epidemic struck, an exposed (E) category was added to the model—resulting in SEIR—to account for the gestation period, where the person was infected but not passing the infection to others.
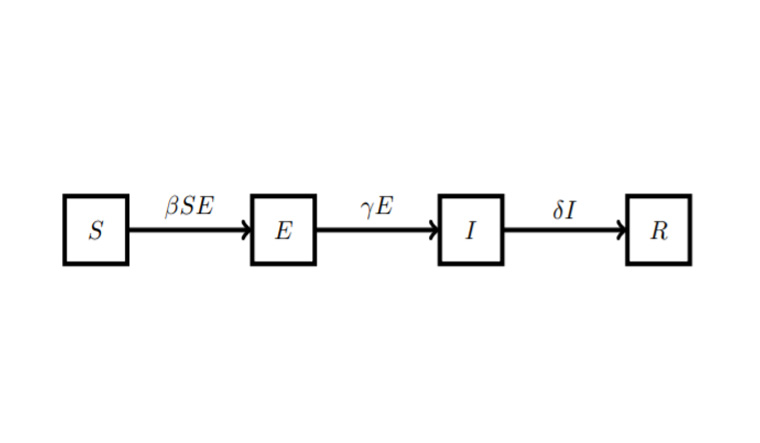
In further years, models like SAIR were developed, to account for possible asymptomatic spreaders. The mechanistic models then evolved into structured metapopulation models, which captured how different demographics mix across populations, and further into agent-based network models.
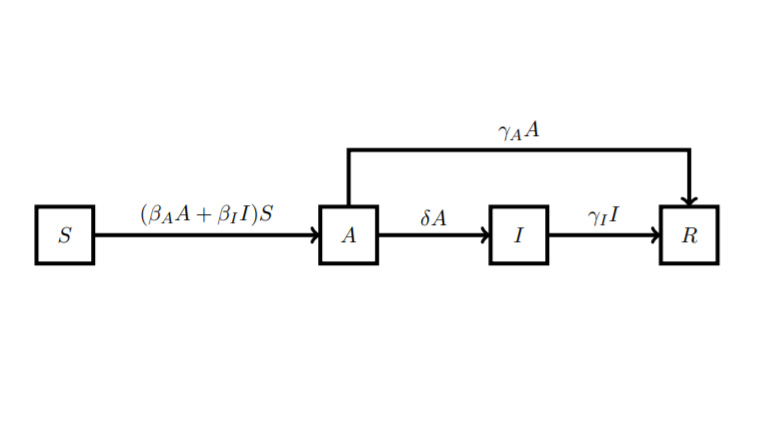
So, where does SUTRA come into play? While the SAIR model was the first one to make a clear distinction between asymptomatic and symptomatic patients, it does make some unrealistic assumptions—SUTRA (in the same SIR family of models) solves for that by replacing the A and I groups with U for 'Undetected but Infected' and T for 'Tested Positive', according to the research paper. There are four compartments in SUTRA—S, U, T, R1, and R2; R1 and R2 denote the group of removed cases from U and T respectively. Here, the susceptible individual (S) become infected, all infected remain undetected (U) for varying periods of time; the undetected then transition into either the asymptomatic removed (R1) category or tested positive (T) and then removed (R2) category.
SUTRA doesn’t take into account the human factor, rise in variants, virus behaviour, or anything of that nature. "We just look for what the data tells us. The data told us that the contact rate came down significantly during lockdown. It was data that told us that contact rate went through the roof in March," said Agrawal.
How does the model work? The innate unpredictability of the novel coronavirus, and the externalities which include state intervention measures, necessitate extra parameters—a total of six, when compared to three in earlier models. Apart from the standard β and γ, there is ε (epsilon, the ratio of detected to total infected) and ρ (rho, or what fraction of the population was affected by the pandemic). If a lockdown is imposed, β changes. If the testing value shifts, ε changes. Once the spread increases, ρ changes.
Changes in these parameters result in phase shifts in the pandemic. The study divides the entire timeline of the pandemic into phases, such that within each phase, the parameters are almost constant. As the phase changes, parameter values drift for a period of time before stabilising—simulations during the drift period can result in erroneous assessments. The rest of duration of the phase is called the stable period—the model can predict the future course of the pandemic as long as the parameter values do not change significantly.
So, how effective has SUTRA been in its predictions? According to the study, SUTRA, on April 29, predicted that the cases would hit the peak between May 4 and 8, peaking at around 390K cases—a very good match; In the month of July, for the US, it indicated a peak in August-end at around 152K infections per day—the actual peak was on September 1 at 166K infections. “The graph equation holds for 62 per cent of the time. The rest is the drift period,” says Agrawal.
Inaccurate data, underreported or incomplete for a plethora of reasons, is a real threat to any model. The spectre only became more pronounced when bodies of coronavirus patients started swelling up all along the Ganges in India. In SUTRA, the possibility of underreporting/missed data is automatically factored in—the model operated on available data. One of the most interesting findings, says Agrawal, was the presence of a very strong mathematical correlation between available data and the actual infections. “It was almost as if what was reported was a scaled down, structured version of reality," he says.
But, SUTRA also has its many critics, some targeting the model for "praising" the Uttar Pradesh government's handling of the pandemic. Tweeted Ashoka University professor Gautam Menon: "The model does not have compartments representing the numbers of hospitalised or numbers of severely ill, all useful quantities if estimates for the numbers of those requiring, say, ICU beds. It is not age-structured, has no contact structure, has far too many parameters that are free to vary, and has as no IFRs in it, so measures of mortality are impossible. Two states with very different population structures, eg one with a low median age [UP] or one with a high one [Kerala] will have very different levels of serious cases as well as of mortality. The SUTRA model has nothing—can have nothing—to say about this."
@MenonBioPhysics gave an good critique of SUTRA model a few days ago (see https://t.co/N69x7hqdgC). It makes many important points regarding epidemiological modeling. In this thread, I am going to develop his analysis further and show what is the purpose of SUTRA model.
— Manindra Agrawal (@agrawalmanindra) October 29, 2021
In an interview with THE WEEK, Agrawal opens up on SUTRA, rebutting some of the criticisms facing the model, on data integrity in India, and evaluating how different states have fared during the pandemic. Edited excerpts:
1. What are the different ways in which mathematical modelling can help us during a pandemic?
Mathematical models, when we talk about pandemic progression, are typically a set of differential equations which try to estimate the trajectory of the disease outbreak. It tries to predict how everyday cases are going to be coming up, how numbers will rise, and so on. That is captured through differential equations. The first modelling was done nearly a hundred years ago, in the time of the Spanish flu [the Kermack-McKendrick SIR model]. Since then, it has become quite popular, and there are multiple variations. But, at the heart, it is the same SIR model which is used to predict the trajectory.
2. What does SUTRA tell us, and what does it not?
Take the case of the parameter beta. What our model will show is that beta has changed. Identifying the underlying cause behind that is something our model is unable to do. To understand that, one has to look to the ground and see what has shifted, and then correlate one to the other. Humans have to do that. What our model can do is flag the change in particular parameters.
3. The efficacy of lockdowns is currently one of the most hotly debated topics related to the pandemic. What does SUTRA data from India say about that?
We did a state-wide analysis of lockdowns during the second wave. In the first wave, there was uniform lockdown across the country, so there was nothing much available to compare. In the second wave, different states adopted different levels of lockdown. Our conclusion from SUTRA analysis is that a very strict lockdown is not substantially better than a medium-level lockdown. [In the latter] People can go to offices and other different places, even when it does not allow indoor crowding activities and such. Lockdowns of all kinds reduce beta. That is a given. What we found though was that the reduction in beta achieved by a medium type of lockdown was as good as the ones achieved by a strict lockdown [where except for emergency services, nothing much is permitted].
4. One of the criticisms against SUTRA was that it failed to consider some of the larger population dynamics, how different demographics interacted with each other, and other questions that could be crucial in interpreting the data.
Having too many parameters introduces more error into the system. Inferring those parameter values becomes too challenging. [The point was that] Different states followed different trajectories, for different reasons. Whichever path they took, they tried to do a good job. Some people criticise Kerala [because of high infection rate], as they also do for other states, but the decisions are taken in good faith. We can follow the pandemic trajectory of the different states, but what we learn from one state cannot be translated into another.
5. Which states, would you say, are the most interesting studies?
The most fascinating one has been Kerala, because of the very long tail of infections that they have been facing. The trade-off for them was that they reduced the peak, but at the cost of having a very long and extended tail. [Should they have gone by that plan?] There are pros and cons to all decisions. They are perhaps the only state to have adopted such a strategy; Maharashtra is perhaps another one, but it is somewhere in between, with a tail that is longish, but not as big as Kerala. Uttar Pradesh is another interesting state. UP and Kerala are both poles apart in their dealing with the pandemic, with UP having adopted a strategy of ‘chasing the pandemic’.
6. Could you elaborate on that a little?
Everybody knows about the Test Positivity Rate (TPR) [the percentage of coronavirus tests that turn out positive], and there is a general understanding that when TPR goes above 10 per cent, the pandemic is spreading very fast. But, can we infer something from the TPR about the testing strategy being used by a state? Not really. Looking at Test Positivity Rate doesn't tell us about testing strategies. So, what we came up with is a Normalised Test Positivity Rate [NTPR], which is TPR divided by the percentage of infected population at the time. If the ratio is close to 1, it means the testing strategy is random. If it is well above 1, the testing strategy is targeted. If NTPR is less than 1, you are testing a large number of people [not just symptomatic ones or susceptible ones], and ‘chasing the pandemic’. In fact, Kerala, in the first year of the pandemic [in 2020], had NTPR way below 1 because they were chasing the pandemic, and were able to control the spread very successfully. But, their strategy later changed from chasing the pandemic to targeted testing. The NTPR for Kerala is now at 3, or somewhere higher, and has been thereabouts for the past several months. So, it was possibly a conscious decision to no longer chase the pandemic, and instead focus on symptomatic cases. In UP, NTPR has been less than 0.5, right from the beginning of the pandemic to this day. That is very fascinating. I never expected it to be that case. The data suggests that the UP has been chasing the pandemic all throughout. That was a very interesting learning.
7. Would you recommend a change in strategy for any state with the data you have in hand?
I am not saying that one strategy is better than the other. I am just observing which state is following which plan.
8. Your model factors in the question of incomplete/inaccurate infection data, with one of the most interesting findings being that the reported data mapped so well to the reality, in a deep, structured relationship. But, will this same phenomenon hold for foreign countries? If we were to try to export SUTRA to the global stage, maybe Latin America or Africa, would the same phenomenon [the strong relation between reported data and reality] hold there?
Absolutely. We have data from these countries, and the same phenomenon is observed there also. Now, the interesting point is, people keeping talking about the poor quality of infection data coming out of India; I invite their attention to the data coming out of countries like the US. There are such jerks in the curve that it is clearly a case of poor reporting. Indian data is so smooth. Sometimes, we assume that just because our systems are not as good as many other countries, we must be doing very badly. But, this is one place where I think we have done better than many other countries.
9. With a surge in Omicron variant cases being reported, what is your outlook?
We did a simulation for South Africa, and found something interesting. The value of beta in South Africa jumped from 0.5 to 1 in the month of August. What this data would strongly suggest, I would say, is that Omicron has been active there from September. And, it could be as twice as infectious as Delta. Because beta multiplied by a factor of 2. Also, this [raises many possibilities]. What if I had run a simulation for South Africa in September? We could have flagged that something strange was happening, with their beta making [an unprecedented] jump to 1. There is a lot of value I see in our kind of modelling that allows us to understand the behaviour of the pandemic, much before it actually shows in numbers. In September, coronavirus numbers in South Africa were still coming down. But, beta jumped.
10. What are your expectations for India?
We are continuously monitoring India, and there has been no jump so far. But there will be a jump. Our expectations are that it won't cause much damage, going by data we have from South Africa.
11. The coronavirus is a phenomenon unlike anything we have seen before. How do the large number of variants affect the core mathematical premises of SUTRA? For instance, does the first basic equation [S+U+T+R1+R2=1] hold when, because of the rise of multiple variants and the threat of immune escape and reinfections, one person can simultaneously be in the recovered [R] category and the susceptible [S] category?
What you have pointed out is true. This is analogous or indistinguishable from the situation where a person is in the recovered category, and a duplicate of this person exists in the susceptible category. Which is basically the same situation where the population has grown. In our model, this is captured by the fact that the reach parameter (ρ/rho) has expanded to include one more person. This makes reach [rho] go beyond one. That is how such a situation gets factored in. The model can capture that and this also tells us what fraction of people have lost immunity because of new mutants.